Case Study: MINING - Getting to Carbon neutral by 2050 starts with making the right technological choices
Summary
Our client is a diversified natural resources company that is engaged in mining and mineral development, including steelmaking, coal, copper, zinc, and energy. As part of their commitment to climate action and responsible resource development, our client set an objective to be carbon neutral across all operations and activities by 2050. Specific to this project, our client’s vision is to reach and exceed its carbon footprint reduction targets via the optimized transition and implementation of a sustainable intelligent transportation system comprised of a combination of electrical trucks, recharging technologies, rail, and conveyor systems. To do so, our client opted to contract out the optimization of an existing AnyLogic simulation model to run as many scenarios as will be required to meet its objectives. This pilot project allowed our client to yield the ambitious results desired and extend to other operations.
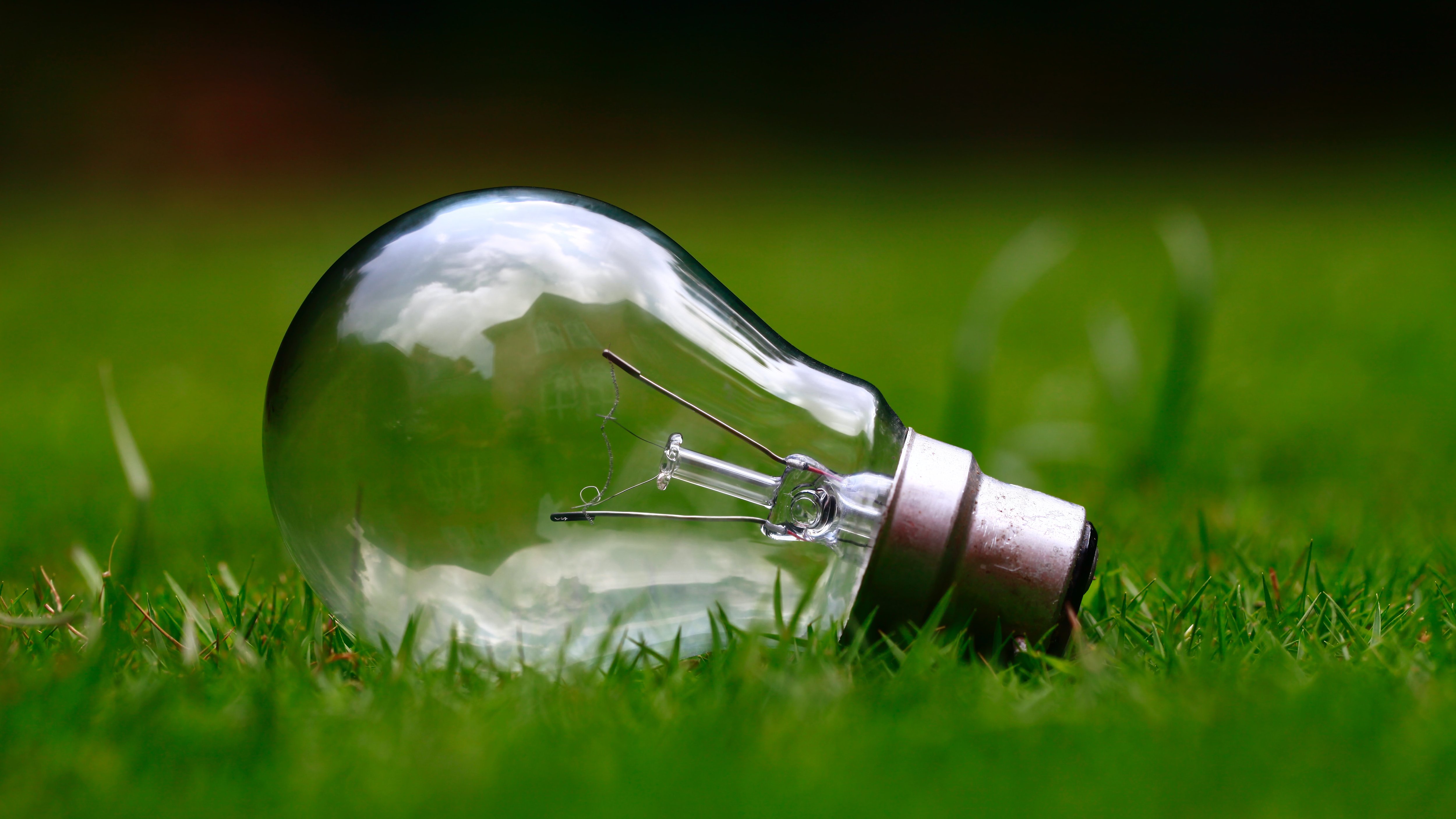
Challenge
This project had three folds as follows:
1) Reducing the run time of the existing high fidelity Anylogic model to below 2 minutes on average per year for a period of 30 years;
2) The successful integration of the Anylogic model with Microsoft Project Bonsai as an AI machine in the loop;
3) The optimization of the combination of electrical trucks, recharging technologies, rail, and conveyor systems that will bring the best return on investment over the next 30 years. The model accounted for the transition from diesel to electrical trucks spanning over multiple years. The acquisition and lifecycle costs were also considered.
Solution
The objective of the first phase was the optimization of the high-fidelity existing AnyLogic model below 2 minutes on average over a period of 30 years by simplifying the model logic. The second phase comprised of the smooth integration of the AnyLogic model output into an AI brain and acceptance of AnyLogic input from the AI brain in the loop results. The last phase of the modeling project related to the selection of the optimized combination of electrical trucks and recharging technologies to achieve carbon reduction footprint targets and minimize the acquisition and operational/lifecycle sustainment costs over a period of 30 years.
The following functionalities were considered as part of this modeling effort:
- Truck transportation time, number of trucks, etc.
- Transition to new electric technology (electrical trucks, re-charging strategies)
- Addition/Removal of trucks
- Loading Unit / Hauling Unit capacity, speed, number, location, etc.
- Maintenance and downtime
For reducing the run time of the existing model, the “scenarios” comprised of deactivating several model functionality logics and studying the resulting impact on run time. For the optimization of the transition to electrical trucks, the scenarios comprised of the model running numerous combinations of different technologies (truck types, number and recharging stations) resulting in the optimization of our key performance indicators, as follows:
- Reduction of Existing model run time
- 2 minutes average model run time over a 30-year period.
- AI brain Integration
- Successful validation of AnyLogic’s integration into the AI brain.
- Transition to Electric Technology
- Optimization of the number and types of electric trucks and charging stations.
Results
The overall consolidation of improvements led to a significantly improved model run time of 9 seconds per year on average, which is a noteworthy reduction from the initial 160 seconds.
The model also provided OpEx and CapEx, yearly GHG emissions, and total haulage for the year to the AI brain after each iteration. This allowed the brain to compare/analyze scenarios leading to the optimization of the solution in terms of costs (external to AnyLogic).
The model outputs were intended for a given diesel truck retirement rate and the introduction rate of the combination of 4 types of electric trucks and 3 recharging technologies. The scenarios were selected by the client. The Anylogic model results were run in the AI brain for the optimization of the selection of electric technology using the model.