As industries embrace the digital transformation of their operations, terms like Digital Twin and Simulation Digital Twin are increasingly common in conversations about optimization and innovation. While these terms are often used interchangeably, they represent distinct concepts with unique capabilities. Understanding the difference is essential for selecting the right tool to meet specific business needs. In this blog post, we’ll explore these differences and clarify how they serve different purposes in the decision-making process.
What is a Digital Twin?
A Digital Twin is a virtual replica of a physical system, asset, or process. It integrates real-time data from sensors, IoT devices, and operational systems to provide a dynamic, up-to-date representation of its physical counterpart. Digital twins are widely used for monitoring, diagnostics, and visualization.
Key Characteristics of Digital Twins:
- Real-Time Monitoring: Digital twins continuously reflect the real-world state of a system, capturing live data to track performance and detect anomalies.
- System Insights: They provide a detailed, holistic view of how a system is performing, often through dashboards or visualizations.
- Reactive and Predictive: While primarily used for monitoring, advanced digital twins may incorporate predictive analytics to forecast issues or failures.
- Focus on Current State: The digital twin is primarily concerned with the "as-is" state of the system, offering insights into what is happening now.
Digital twins excel at providing visibility into complex systems, enabling stakeholders to monitor operations, identify inefficiencies, and respond to problems as they arise. However, they lack the simulation-driven capability to test "what-if" scenarios or explore alternative strategies in a dynamic, virtual environment.
What is a Simulation Digital Twin?
A Simulation Digital Twin builds upon the foundation of a digital twin by incorporating a Simulation Model. While it shares the ability to reflect real-time data, a simulation digital twin uses this data to predict outcomes, evaluate scenarios, and recommend optimal decisions. This combination transforms a digital twin from a monitoring tool into a dynamic decision-making platform.
Key Characteristics of Simulation Digital Twins:
- Integrated Simulation Models: At its core, a simulation digital twin includes a model of the physical system that replicates its behavior. This model allows the twin to simulate future outcomes based on real-time inputs.
- Scenario Testing: Simulation digital twins can answer "what-if" questions, enabling users to explore the potential impact of decisions or disruptions before implementing changes in the real world.
- Real-Time Predictions: By combining live data with simulation capabilities, the twin predicts how the system will behave under specific conditions, offering prescriptive insights.
- Decision Support: A simulation digital twin is not only a mirror of the current system but a forward-looking tool for optimizing operations and mitigating risks.
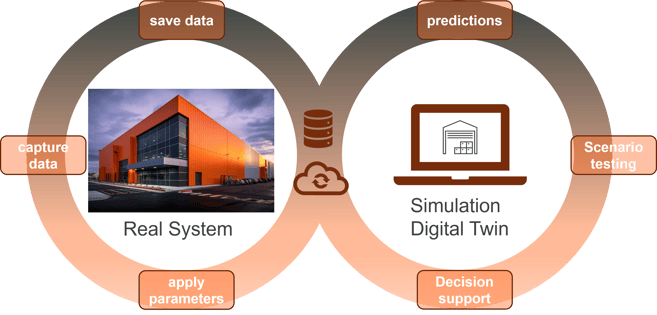
The simulation capability of this type of twin makes it indispensable for tactical and operational decision-making, particularly in environments where real-time adjustments are critical to success.
Key Differences Between a Digital Twin and a Simulation Digital Twin
Aspect |
Digital Twin |
Simulation Digital Twin |
Simulation Capability |
None |
Core feature for scenario testing and optimization |
Key Questions Addressed |
What is happening right now? |
What will happen if we do X? |
|
Are there anomalies or inefficiencies? |
What is the best course of action? |
Decision-Making Focus |
Reactive and predictive |
Proactive, predictive and prescriptive |
Real-Time Data Integration |
Yes |
Yes |
Scenario Testing |
No |
Yes |
Time Horizon |
Immediate (current state of the system) |
Near-future (short-term predictions) |
Why Add Simulation to a Digital Twin?
A standard digital twin provides a robust foundation for monitoring and understanding a system’s real-time state. However, modern industries face increasingly complex challenges that require not just visibility into current conditions but also the ability to predict and act proactively. Adding simulation to a digital twin unlocks capabilities that address these challenges:
- Enhanced Decision-Making: Simulation digital twins enable organizations to evaluate multiple courses of action, select the optimal one, and implement it with confidence.
- Mitigating Risk: By simulating potential disruptions or failures, businesses can develop contingency plans and prevent costly mistakes.
- Optimizing Operations: Simulation digital twins can analyze real-time data to suggest improvements, such as adjusting production schedules, optimizing resource allocation, or responding to supply chain disruptions.
- Short-Term Tactical Insights: While a digital twin focuses on monitoring, a simulation digital twin thrives in environments where tactical agility and responsiveness are crucial.
The Role of Real-Time Data
Both digital twins and simulation digital twins depend on accurate, real-time data to reflect the state of the physical system. However, the way they use this data differs:
- Digital Twins: Use real-time data to monitor and visualize the system’s current performance. Insights are primarily descriptive, providing a clear picture of what is happening now.
- Simulation Digital Twins: Use real-time data to initialize the simulation model and predict future outcomes. This capability enables prescriptive and proactive decision-making, which goes beyond monitoring to support operational optimization.
Conclusion:
Both digital twins and simulation digital twins are valuable tools in the digital transformation toolbox, but they serve different purposes:
- A Digital Twin provides a real-time mirror of a system, empowering organizations to monitor and respond to current conditions.
- A Simulation Digital Twin adds the ability to predict and optimize, offering powerful decision-support capabilities that go beyond monitoring to actively shape outcomes.
Choosing between these tools—or integrating both—depends on your business objectives. If your goal is to maintain real-time visibility, a digital twin may suffice. However, if you want to explore "what-if" scenarios, predict outcomes, and optimize operations, a simulation digital twin is the more advanced solution.
Are you ready to unlock the power of simulation digital twins for your organization? Let’s start the conversation!
Send me a note at aouellet@simwell.io
Alexandre Ouellet, CEO at SimWell